基于多标签学习的异质信息网络数据挖掘的设计(硕士)
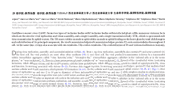
基于多标签学习的异质信息网络数据挖掘的设计(硕士)(论文23000字)
摘要
现实世界中的许多复杂系统可以形式化为网络,其中节点表示对象,链接表示对象之间的交互。这些网络大部分是异质的,包含各种类型的对象和关系。例如,在线社交网络Twitter包含关于诸如用户,位置和推文之类的节点的类型的信息,具有诸如发推文/转发推文,关注/跟随,签入/登记等链接的类型。
作为链接挖掘中的一个关键问题,链接预测的目的是基于当前或历史网络来预测未来链接的形成[1]。最近,在异质信息网络中由于其更广泛的应用前景推动了链接预测的巨大兴趣[2]。
在这种情况下,我们提出了两种新的关系预测方法MULRP(基于多标签学习的链接预测器)和MUILP(基于多实例的链接预测)。MULRP方法首先使用元路径的概念来定义节点之间的关系,然后将关系信息集成到多标签学习框架下的预测模型[4]。最终的模型可以预测新的目标链接/关系以及揭示关系(即标签)的相关性。这是第一个将多标签学习引入链接挖掘的工作。这里,标签是指由元路径定义的关系的类型。而且,该方法可以为如何形成新的链接提供建议,而不仅仅是扮演预测者的角色。MUILP则是多实例学习在链接预测方向的尝试,实验结果表明,MUILP的效果高于传统的逻辑回归但是低于MULRP。 [资料来源:http://doc163.com]
关键词:链接预测,异质网络,多标签,社会网络分析
Abstract
Many complex systems in the real world can be formalized into networks, where nodes represent objects and links represent interactions between objects. Most of these networks are heterogeneous, containing various types of objects and relationships. Online social network Twitter, for example, contains about such as a user, location, and tweets nodes such as the type of information, such as sending tweets/forwarding tweets, attention/follow, check in/registration link types.
As a key problem in link mining, the purpose of link prediction is to predict the formation of future links based on current or historical networks [1]. Recently, due to its wider application prospect in heterogeneous information network, the great interest of link prediction has been promoted [2].
In this case, we propose two new relationship prediction methods, MULRP (Link Predictor Based on Multi-Label Learning) and MUILP (Link Prediction Basedon Multi-Instance). The MULRP method first uses the concept of a meta-path to define the relationships among nodes and then integrates the relational information into a predictive model under a multi-label learning framework [4]. The final model predicts the new target link / relationship and reveals the relevance of the relationship (ie, the label). This is the first effort to introduce multi-label learning into link mining. Here, the tag refers to the type of relationship defined by the meta-path. Moreover, this approach can provide advice on how to form a new link, not just acting as a forecaster. MUILP is a multi-instance attempt to learn the direction of link prediction. The experimental results show that MUILP is superior to traditional logistic regression but lower than MULRP.
Key words:Link Prediction,Heterogenous Network,Multi-label,Social network analysis
目录
第一章绪论 1
1.1 研究背景和意义 2
1.2 研究现状 2
1.3 研究难点和挑战 4
1.4 本文研究内容 5
第二章异质信息网络链接预测问题 6
2.1 异质信息网络分析 6
2.1.1 同质信息网络 6
2.1.2 异质信息网络 6
2.1.3 基于元路径的分析方法 8
2.2 链接预测 10
2.2.1 同质信息网络中的链接预测 11
2.2.2 异质信息网络中的链接/关系预测 13
2.2.3 基于监督学习的链接预测方法 14
2.2.4 评价指标 14
2.3 本章小结 15
第三章基于多标签学习的链接预测器 16 [资料来源:http://www.doc163.com]
3.1 多标签学习 16
3.1.1 多标签学习方法 17
3.1.2 评价指标 18
3.2 本章算法基本思想 20
3.3 特征提取 20
3.1.1 同质网络中基于近邻的特征 20
3.1.2 异质网络基于元路径的特征 22
3.4 算法伪代码 22
3.5 本章小结 24
第四章基于多实例学习的链接预测 25
4.1 多实例学习 25
2.4.1 多实例学习 26
2.4.2 多实例学习算法 26
4.2 本章算法基本思想 27
4.3 本章小结 27
第五章实验与分析 28
5.1. 实验数据集 28 [来源:http://Doc163.com]
5.2. 对比方法设置 29
5.3. 实验设置 30
5.4. 实验结果与分析 31
5.4.1 MULRP方法的结果与分析 31
5.4.2 MUILP方法的结果与分析 32
第六章总结与展望 33
参考文献 35
致谢 39
上一篇:平板探测器的图像性能优化(硕士)