一种互信息的逼近方法
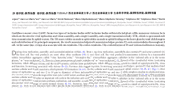
一种互信息的逼近方法(中文4100字,英文PDF)
FUMIO HIAI 1 AND D´ENES PETZ 2
摘要: .一个新的表达式如用一个特定的渐近界限经由“不连续的微观状态” 排列来提供连续的和不连续的随机变量的互信息。
[资料来源:https://www.doc163.com]
引言
在信息论中我们知道随机变量x、y是互信息上的两个很重要的量。随着x、y的不同定义有不同的意义。当x、y是连续变量时,我们根据Boltzmann-Gibbs热力学函数H(•)就有如下表达式:
而当x、y是不连续的变量时,上述的H(•)就被Shannon热力学之函数所代替,一种更为实际和更严格的定义就出来了,此时平均信息量热力学之函数表达为:
式中μ(X,Y)是(X,Y)的联合分布,µX µY分别是X,Y的分布。
在此写这篇论文的目的就是在于指出互信息I(X Y)在某些方式下改变(X,Y)的近似连接组成的“不连续的微观状态”量而得到一个特定的渐近界限。在论文的第一部分,在通常情况下我们考虑n为任意的一组有限变量(X1, ..., Xn),表示为 Δ(X1, ..., Xn; N, m, δ)。(x1, ..., xn) 中的xi N ,它在瞬间连接点上(在n的相同分布点上)使得m近似等于(X1, ..., Xn)。误差为δ。此外,对于由σi SN组成的(σ1, ...,σn)排列我们记作Δsym(X1, ..., Xn; N, m, δ)。就如 (σ1(x1), ...,σn(xn)) Δ(X1, ..., Xn; N, m, δ) 中的x1, ..., xn 。当 在 向量中按次序增长时,我们可得渐进量为:
[来源:http://www.doc163.com]
在相同的概率量下 在SN中表示聚集lim (或者lim ),然后由
, \0得到:
在1 i n时H(Xi) >- 。这种情况下我们可以得到一种离散化的互信息集合(或排列)。
按照上面的这种方法,我们可以把它应用到(X1, ..., Xn)中不连续的任意离散变量n中去 。但是 集合的精确度在不连续微观状态时和连续型时的连续变量是稍微有些不同的。我们第一部分讨论的是连续变量的情况。对于不连续型的我们在第二部分有个别提及到。(毕业设计)
这个想法在自由互信息推动原理的第六章的第三页有提起。一个类似于Voiculescu’s 的方法用来改进自由平均互信息量。而这个自由平均信息量是自由概率Boltzmann-Gibbs平均信息量的近似量。向量 和对称集合 SN被厄密共轭矩阵N × N和一元集合 U(N), 分别代替。 这样,这种离散方法在这里的某些意义上讲是一种正常的轨迹逼近方法。
A NEW APPROACH TO MUTUAL INFORMATION
FUMIO HIAI 1 AND D´ENES PETZ 2
Abstract. A new expression as a certain asymptotic limit via “discrete micro-
states” of permutations is provided to the mutual information of both continuous [资料来源:Doc163.com]
and discrete random variables.
Introduction
One of the important quantities in information theory is the mutual information of
two random variables X and Y which is expressed in terms of the Boltzmann-Gibbs
entropy H(·) as follows:
I(X ∧ Y ) = −H(X, Y ) + H(X) + H(Y )
when X, Y are continuous variables. For the expression of I(X∧Y ) of discrete variables
X, Y , the above H(·) is replaced by the Shannon entropy. A more practical and rigorous
definition via the relative entropy is
I(X ∧ Y ) := S(μ(X,Y ), μX ⊗ μY ),
where μ(X,Y ) denotes the joint distribution measure of (X, Y ) and μX ⊗μY the product
of the respective distribution measures of X, Y .
The aim of this paper is to show that the mutual information I(X∧Y ) is gained as a
certain asymptotic limit of the volume of “discrete micro-states” consisting of permutations
approximating joint moments of (X, Y ) in some way. In Section 1, more generally [资料来源:http://doc163.com]
we consider an n-tuple of real bounded random variables (X1, . . . ,Xn). Denote by
(X1, . . . ,Xn;N,m, ) the set of (x1, . . . , xn) of xi ∈ RN whose joint moments (on the
uniform distributed N-point set) of order up to m approximate those of (X1, . . . ,Xn) up
to an error . Furthermore, denote by sym(X1, . . . ,Xn;N,m, ) the set of (1, . . . , n)
of permutations i ∈ SN such that (1(x1), . . . , n(xn)) ∈ (X1, . . . ,Xn;N,m, ) for [资料来源:http://www.doc163.com]