基于卷积神经网络的手写数字识别
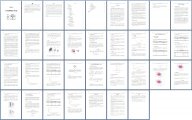
基于卷积神经网络的手写数字识别(任务书,开题报告,论文12800字)
摘要
手写数字识别技术是自动识别手写体阿拉伯数字识别技术或计算机的光学模式识别技术的一个分支标志。数字手写体文字识别技术有着广泛的应用,如邮政编码,财务报表,税务统计系统、银行票据等。此方面的全球需求正处于增长中,自动化技术应用在数字手写体识别上的要求迫在眉睫。
传统的识别方法普遍存在精度低、效率低下等问题,本文使用了深度学习领域广泛使用的卷积神经网络以及谷歌公司所提供的tensorflow框架来实现手写数字识别应用,在遵循LeNet-5模型结构的基础上,通过引入指数衰减学习率、滑动平均、正则化等方法来优化模型并增强其的泛化能力,防止出现过拟合的现象,便于实现更全面的模型应用。本文使用MNIST数据集进行训练和测试,分类的准确率可以达到95%以上,实验结果证明本文所设计模型具备良好的分类性能与实用价值。
关键词:手写数字识别;卷积神经网络;滑动平均
Abstract
Handwritten digit recognition technology is a branch symbol of automatic recognition of handwritten Arabic numeral recognition technology or computer optical pattern recognition technology. Digital handwritten text recognition technology has a wide range of applications, such as postal codes, financial statements, tax statistics systems, bank notes and so on. Global demand in this area is growing, and the requirements for the application of automation technology in handwritten digit recognition are imminent.
The traditional recognition method has the problems of low precision and inefficiency, this paper uses the convolution neural network widely used in the field of deep learning and the TensorFlow framework provided by Google Company to realize the application of handwritten digital recognition, on the basis of following the structure of LeNet-5 model, by introducing exponential attenuation learning rate, Sliding average, regular and other methods ,we optimize the model and enhance its generalization ability, to prevent the occurrence of fitting phenomenon so as to achieve a more comprehensive model application. In this paper, we use MNIST DataSet for training and testing, the accuracy of classification can reach more than 95%, the experimental results show that the model designed in this paper has good classification performance and practical value.
Keywords: handwritten digital recognition; convolution neural network; sliding average
[资料来源:http://Doc163.com]
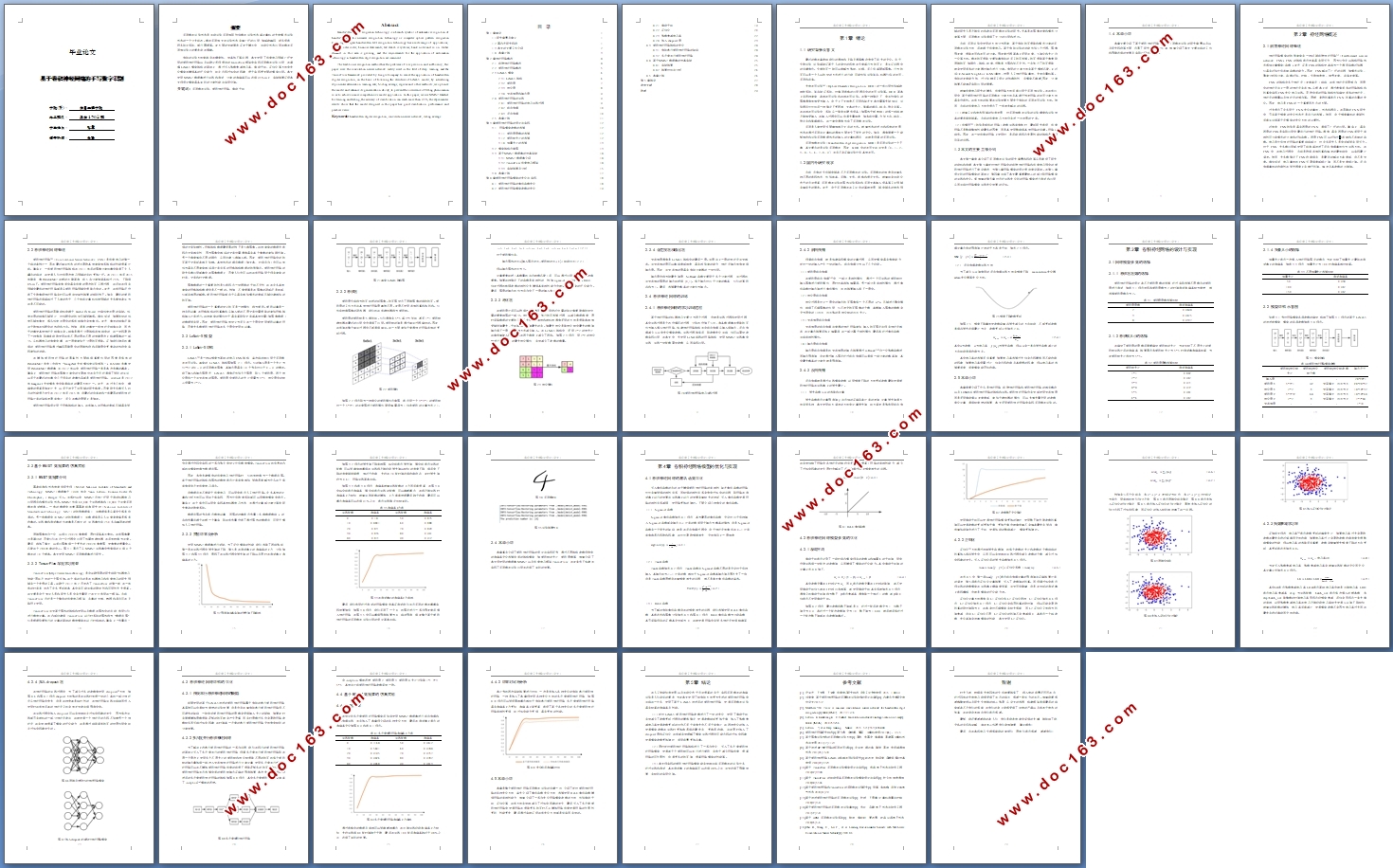
目 录
第1章绪论 1
1.1 研究背景及意义 1
1.2国内外研究现状 1
1.3本文的主要工作介绍 2
1.4 本章小结 3
第2章神经网络概述 4
2.1 前馈神经网络概述 4
2.2 卷积神经网络概述 5
2.3 LeNet-5模型 6
2.3.1 LeNet-5结构 6
2.3.2 卷积层 7
2.3.3 池化层 8
2.3.4 全连接层和输出层 9
2.4 卷积神经网络的训练 9
2.4.1 卷积神经网络的学习训练过程 9
2.4.2 前向传播 10
2.4.3 反向传播 10 [版权所有:http://DOC163.com]
2.5 本章小结 11
第3章卷积神经网络的设计与实现 12
3.1 网络模型参数的选择 12
3.1.1 卷积层层数的选择 12
3.1.2 卷积核大小的选择 12
3.1.4 批量大小的选择 13
3.2 模型结构示意图 13
3.3 基于MNIST数据集的仿真实验 14
3.3.1 MNIST数据集介绍 14
3.3.2 TensorFlow深度学习框架 14
3.3.3 实验结果及分析 15
3.4 本章小结 17
第4章卷积神经网络模型的优化与实现 18
4.1 卷积神经网络的激活函数优化 18
4.2 卷积神经网络模型参数的优化 19
4.2.1 滑动平均 19
4.2.2 正则化 20
4.2.3 指数衰减学习率 22
4.2.4 加入dropout层 23
4.3 卷积神经网络结构的优化 24 [来源:http://Doc163.com]
4.3.1 传统串行卷积神经网络的缺陷 24
4.3.2 多尺度并行卷积神经网络 24
4.4 基于MNIST数据集的仿真实验 25
4.4.1 实验结果 25
4.4.2 结果对比与分析 26
4.5 本章小结 26
第5章结论 27
参考文献 28
致谢 29