基于生成对抗网络的多源医学影像融合算法研究
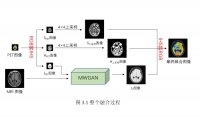
基于生成对抗网络的多源医学影像融合算法研究(任务书,开题报告,论文16000字)
摘要
医学图像融合技术是当今图像融合技术的一大重要应用,其在许多生物医学研究和临床应用例如医学诊断、检测和治疗中扮演着举足轻重的角色,为医用的后续处理和决策执行提供了关键的信息,尤其是异分辨率医学图像的融合也为医学应用场景的多样性及复杂性提供了更多的支持和保障。现有的医学图像融合方法需要手动设计复杂的活动水平测量和融合规则,这受到了实施难度和计算成本的限制。此外,由于升级硬件设备和算法的难度,提高其中一张源图像的分辨率或降低另一张源图像的分辨率以融合异分辨率源图像的策略必然导致源图像部分信息的含糊或丢失,还可能产生一些冗余信息。而且,源图像的信息在融合图像的比例也难以调节。因此,对异分辨率医学图像的融合研究具有重要意义。
本文提出了一种新型的基于wasserstein生成对抗网络(WGAN)的端到端模型,用于融合异分辨率磁共振成像(MRI)医学图像和正电子发射断层扫描(PET)医学图像,该算法称为MWGAN,比基于生成对抗网络的算法更加稳定,此算法通过在生成器和两个判决器之间建立两个对抗性竞争,旨在使融合图像同时具有来自高分辨率MRI图像中器官软组织结构细节(纹理细节信息)以及来自低分辨率的PET图像中功能和代谢信息(像素强度信息)。
为实现深度学习覆盖整个融合过程,本文使用反卷积操作,通过训练自动获得参数,以融合异分辨率源图像,避免源图像信息丢失及冗余信息产生。并且本文也提出了一种新的损失函数设置策略来有效地调整融合图像中源图像中的信息。
在公开数据集与其它五种算法的直观定性比较证明了本文提出的MWGAN算法优于现有融合算法;在定量8项指标比较中,EN、MG、EI、PSNR、CC和SF这6种指标分别达到了6.0096、0.0519、0.4085、63.5816、0.8694及0.1418,在这六种算法中均排第一,其余两项指标SD和SSIM也分别达到了0.3582及1.9883,在这六种算法中均排第二。此外,MWGAN也适用于异分辨率的MRI医学图像和CT医学图像的融合,与现有技术相比,它也达到了很好的融合效果。
关键字:医学图像;WGAN;端对端;异分辨率
Abstract
Medical image fusion technology is an important application of today's image fusion technology. It plays a pivotal role in many biomedical research and clinical applications such as medical diagnosis, detection and treatment, providing key information for medical subsequent processing and decision-making.In particular, the fusion of different-resolution medical images also provides more support and guarantee for the diversity and complexity of medical application scenarios.Existing medical image fusion methods require manual design of complex activity level measurement and fusion rules, which is limited by implementation difficulty and computational cost. In addition, due to the difficulty of upgrading hardware devices and algorithms, the strategy of increasing the resolution of one source image or reducing the resolution of the other source image to fuse the source images of different resolutions will inevitably lead to the loss of partial information of the source image, and possibly generate some redundant information. The ratio of source images information in the fused image is also difficult to adjust.Therefore,it is of great significance to study the fusion of medical imagesofdifferentresolutions.
In this thesis, we proposed a novel end-to-end model based on wasserstein generative adversarial networks which is more stable than generative adversarial networks, termed as MWGAN, for fusing magnetic resonance imaging (MRI) medical image and positron emission tomography (PET) medical image of different resolutions. Our method establishes two adversarial games between a Generator and two Discriminators, aiming to generate a fused image with the details of soft tissue structures in organs (texture details information) from high-resolution MRI image and the functional and metabolic information (pixel intensity information) from low-resolution PET image.
This thesis also uses the deconvolution operation to automatically obtain parameters through training to fuse the source images of different resolutions to avoid information loss without redundant information generated, getting rid of the constraints of traditional methods.And the information from the source images can be effectively adjusted with a new loss function setting strategy provided. [资料来源:http://doc163.com]
The qualitative comparison withotherfivealgorithms on publicly available datasetsdemonstrates the superiority of our MWGAN over the state-of-the-arts; Among the quantitative metrics, the six metrics of EN, MG, EI, PSNR, CC and SF reach 6.096, 0.0519, 0.4085, 63.5816, 0.8694 and 0.1418, respectively, ranking first among the six algorithms. The other two metrics SD and SSIM also reach 0.3582 and 1.9883, respectively, ranking second among the six algorithms. Furthermore, our MWGAN is applied to the fusion of MRI image and CT image from different resolutions, which also achieves an better performance compared with the state-of-the-arts.
Key words:medicalimage; WGAN;end-to-end; different resolutions
课题主要工作内容与论文结构
本文研究的是基于生成对抗网络的多源医学影像融合算法,先从国内外基于深度学习的图像融合算法现状分析其中的不足,再由此提出解决这些不足的新算法,通过实验得出此算法与其他算法的对比表现,并由此扩展到MRI与CT图像的融合[14][15],最后进行总结。
本文的主要工作内容体现在以下三个方面:首先,针对医学图像的融合提出一种基于WGAN[16]的更加稳定的融合算法MWGAN,并提供一种新的损失函数设定策略。第二,实现MWGAN端到端的模型设计,并在整个融合过程中实现深度学习的全覆盖,通过与现有先进融合方法的定性和定量比较,得出本文提出的MWGAN与其他算法相比针对异分辨率MRI和PET医学图像融合表现。最后,通过实验验证本文的MWGAN在MRI和CT医学图像的融合表现。 [资料来源:http://doc163.com]
由此,本文分为五部分来阐述,具体安排如下:
第1章,绪论。对文章的研究背景、研究目的进行介绍,然后进行文献综述,主要介绍国内外学者的研究成果与研究结论以及相关理论的发展现状,最后阐述本文的重要工作与论文结构。
第2章,介绍神经网路、GAN的相关背景知识及MWAGN所基于的WGAN网络框架。
第3章,介绍MWGAN算法。主要针对MRI与PET图像的融合从整个融合过程、MWGAN训练过程、损失函数以及网络结构等方面对MWGAN算法进行全方面介绍。
第4章,实验分析。此章展示了本文的MWGAN算法在大量MRI和PET图像对中定性视觉效果和定量指标方面的表现并与其他最先进的融合算法进行定性及定量对比,以及在MRI和CT图像对的定性比较。
第5章,对文章进行总结及展望。此章对本文的工作做出总结,对异分辨率医学图像的融合工作做出展望。
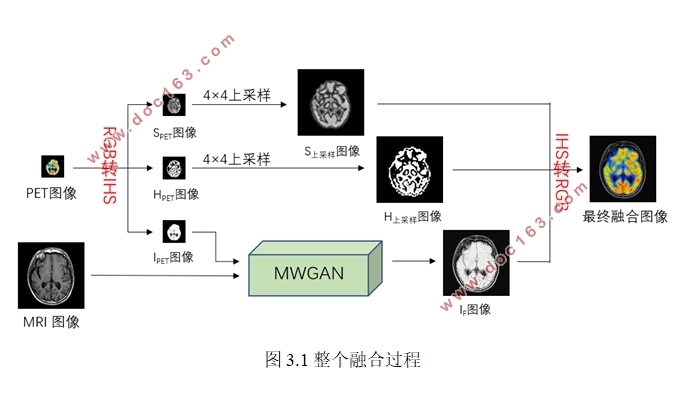
目录
第1章绪论 1
1.1 课题研究背景 1
1.2 基于深度学习的图像融合方法国内外研究现状 2
1.3 课题重要工作贡献与论文结构 3
1.4 本章小结 4
第2章神经网络 4
2.1 神经网络简介 5
2.1.1 神经网络 5
2.1.2 卷积神经网络(CNN) 5
2.2 GAN 6
2.3 WGAN 7
2.4 本章小结 7
第3章 MWGAN算法 8
3.1 图像全局融合过程设计 8
3.2 MWGAN训练过程设计 9
3.3 损失函数设计 10
3.4 网络结构设计 11
3.4.1 生成器网络结构 12
3.4.2 判决器网络结构 13
3.5 本章小结 13
第4章实验设计与结果分析 14
4.1 数据集的选取与处理 14
4.2 训练细节处理 14
4.3 MWGAN算法针对MRI与PET图像的融合结果 15
4.3.1 实验结果定性比较 16
4.3.2 实验结果定量比较 18
4.3.3 生成器参数的剪切分析 22
4.4 MWGAN算法针对MRI与CT图像的融合结果 23
4.5 本章小结 25
第5章总结与展望 26
5.1 本文工作总结 26
5.2 展望 26
参考文献 28
致谢 30
[资料来源:www.doc163.com]