基于深度学习的直流电能质量评价
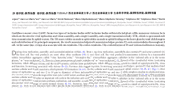
基于深度学习的直流电能质量评价(任务书,开题报告,论文19000字)
摘 要
随着可再生能源的发展,直流电能在我们的生活中发挥着越来越大的作用。本文基于人为定义指标的评价方法与基于信号特征自动提取的方法对直流电能质量进行评估,其中人为定义指标的分类器是模糊层次分析法(Fuzzy Analytical Hierarchy Process,FAHP)与变异系数法的组合,基于信号特征自动提取的分类器是经验模态分解(Empirical Mode Decomposition, EMD)和一维卷积神经网络(1-D CNN)的组合。
以格力光伏小屋的数据做实验,结合深度学习解决复杂问题的能力,搭建一种综合性直流电能质量评价模型,得到准确的结果。首先,利用模糊综合评估法对直流电能质量做出评价,验证方法的可行性;其次,利用上述方法给数据打标签;最后,采用残差网络与循环神经网络的组合结构对数据进行训练,调整网络。
实验结果表明,模糊层次分析法与变异系数法的组合能对直流电能质量做出很好的评估,与我们的预期结果一致。利用经验模态分解得到的数据作为输入,采用残差网络与循环神经网络的组合结构训练数据,准确率非常高。
本文的特色为将用于评估电能质量的传统方法与神经网络相结合,对直流电能质量进行评估。与此同时,还平衡了主观赋权法取决于专家评分和客观赋权法依赖数据的缺点,给出合理的权重。 [资料来源:http://doc163.com]
关键词:模糊层次分析法;一维卷积神经网络;经验模态分解;直流电能质量
Abstract
With the development of renewable energy, DC power plays a more and more important role in our daily life. In this paper, the evaluation method based on defined indicators artificially and the way based on automatic extraction of signal features, which aims to evaluate DC power quality. The classifier of defined indicators artificially is a combination of Fuzzy Analytical Hierarchy Process (FAHP) and coefficient of variation method. The classifier based on automatic extraction of signal features is a combination of Empirical Mode Decomposition (EMD) and OneDimensional Convolutional Neural Network (1-D CNN).
Experiment with the data of Gree Photovoltaic Hut, then combined with the ability of deep learning to solve complex problems. At last, a comprehensive DC power quality evaluation model will be bulid, which can get accurate results. Firstly, using fuzzy comprehensive evaluation method to evaluate DC power quality and verify this method’s easibility. Secondly, the above method is used to label the data. Finally, this combination structure of residual network and cyclic neural network is used to train the data and adjust the network.
Results show that the combination of fuzzy analytic hierarchy process and coefficient of variation method can make a good evaluation of DC power quality, which is consistent with our expected results.Using the data obtained by empirical mode decomposition as input, meanwhile, training data is combined with residual network and cyclic neural network, and the accuracy is very high.
The paper features a combination of traditional methods for assessing power quality and neural networks to evaluate DC power quality. At the same time, it also balances the shortcoming of subjective weighting method depending on expert scoring and objective weighting method relying on data quality heavily, and give reasonable weights finally.
Key Words: FAHP; 1-D CNN; EMD; DC power quality
目 录
第1章 绪论 1
1.1 课题背景及意义 1
1.2 国内外研究现状 2
1.3 论文的内容及章节安排 3
第2章 直流电能质量的相关理论基础 4 [来源:http://www.doc163.com]
2.1 电能质量的定义 4
2.2 电能质量各项指标的定义 4
2.2.1 电压偏差 4
2.2.2 电压波动 5
2.2.3 电压纹波系数 5
2.2.4 电流纹波系数 6
2.2.5 电压暂降 6
2.2.6 短时中断 7
2.3 电能质量综合评估方法 7
第3章 基于FAHP的直流电能质量评估 9
3.1 数据预处理 9
3.2 主观赋权法 9
3.3 客观赋权法 13
3.4 指标隶属度计算 14
3.5 模糊综合评估 15
3.6 实验结果与分析 16
第4章 基于神经网络的直流电能质量综合评估 19
4.1 经验模态分解 19
4.2 深度学习基本知识 20
4.2.1 神经网络基础知识 21
4.2.2 典型神经网络 22
4.3 系统网络结构 23
[资料来源:www.doc163.com]
4.4 实验结果与分析 25
4.4.1 模态分解方法对比 25
4.4.2 不同卷积神经网络对比 27
第5章 总结与展望 30
5.1 工作总结 30
5.2 未来展望 30
参考文献 32
附 录 34
致 谢 43 [来源:http://www.doc163.com]