电力系统负荷预测及其分析
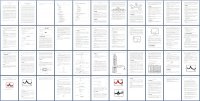
电力系统负荷预测及其分析(任务书,开题报告,外文翻译,论文20000字)
摘要
电力系统负荷预测己成为实现电力系统管理现代化的重要研究内容之一,尤其是短期负荷预测,在电力系统的生产和运行中发挥着重要作用。未来某一时刻的负荷,通常与过去的负荷水平、当前的运行状况、预测期的气象因素以及日期类型等密切相关。但是,当前电力系统行业的短期负荷预测普遍存在着预测方法单一,没有经过模型比较来确定适合于某地区的最佳预测方法,不能精确反映未来负荷走势。针对上述问题,本文做了如下研究工作:
首先,对电力系统短期负荷预测方法进行了回顾,为了验证气象因素对预测水平的影响,以安顺市电力系统为例,根据负荷数据特点及其影响因素,建立两种人工神经网络预测模型。仿真结果表明,考虑天气因素影响后的模型预测精度要高于只考虑历史负荷影响模型的预测精度。
其次,针对BP神经网络预测模型中传统BP算法预测精度不高,收敛速度慢的缺点,采用改进的Lenvenberg-Marquardt(L-M)算法,对短期负荷预测模型进行了重建,并通过仿真验证了改进算法后的模型与基于传统算法的BP神经网络模型相比,训练速度显著提高,能迅速收敛并达到预测精度要求。
最后,由于神经网络对训练负荷样本要求比较高,如果选择的样本没有反映负荷的变化,则预测精度反而会降低。针对这一现象,综合考虑各阶段负荷变化是否受气象因素的影响,建立小波—BP神经网络组合模型。仿真结果表明:组合模型运用于电力系统短期负荷预测,是对单一模型与单一算法的更进一步的改进和优化,预测精度高,预测效果好。验证了理论的正确性和各种方法的有效性、差异性。 [资料来源:http://www.doc163.com]
关键词: 电力系统 短期电力负荷预测 人工神经网络 小波分析组合预测
The analysis and forecasting of power load
ABSTRACT
Power system load forecasting has become one of the important research components of the power system modernization. Especially, the short-term load forecasting plays an important role in the production and operation of the power system. Load of next period is closely related to historical load,current operation status, meteorological factor of forecasting period and the type of date. However, the unitary of the short-term load forecasting methods exists universally in the current power system industry. Model which is no comparison between other models is used for a particular area to determine the best forecasting methods can not accurately reflect the trend of the future load. To deal with the above problems, the paper does the following work:
Firstly, the methods used to forecast the power system load are reviewed. To verify the effect of weather factors to the level of forecasting, taking An Shun city's power system for example, two classes of artifical neural network model are established based on the characteristic of load data. Comparison is carried out between the two models, and the results show that the model considering the weather factors has a higher accuracy than another. [资料来源:http://Doc163.com]
Secondly, to deal with the disadvantages of low accuracy and slow rate of convergence in traditional BP neural network model, the improved Lenvenberg-Marquardt(L-M) algorithm is proposed. Comparison with traditional BP neural network model, simulation results illustrate that the model established based on L-M has a higher speed of training and a faster rate of convergence.
Finally, because the neural network training load samples require relatively high, if weather factors and the samples chosen can not reflect the variation of load, then the prediction accuracy will be reduced. To deal with the phenomenon, considering the changes of load and the effect of weather, the model was established based on the combination of wavelet and neural network. The results show this combined model can get more precise result and better effect it is optimized and improved compared with single model or algorithm in the short-time load forecasting of power system land it testify the right of theory and the effective method.
[资料来源:http://Doc163.com]
Key words: Network(ANN);Power System;Short-Term Load Forecasting Wavelet Analysis ;Combination Forcasti
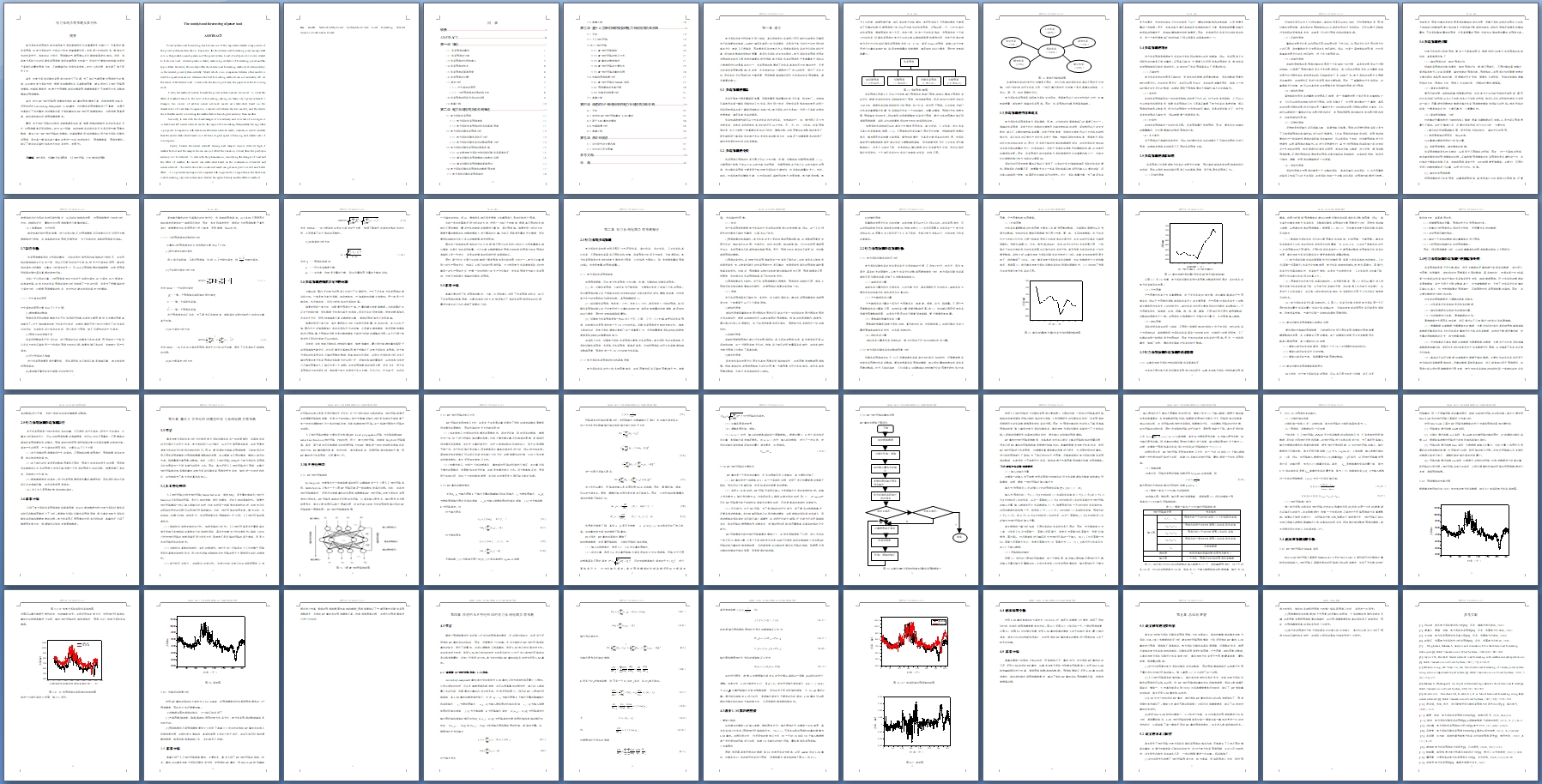
目 录
摘要 I
ABSTRACT II
第一章 绪论 1
1.1 负荷预测的概念 1
1.2 负荷预测的分类 1
1.3负荷预测的作用和意义 3
1.4负荷预测的特点 4
1.5负荷预测的基本原理 4
1.6负荷预测的步骤 6
1.7误差分析 7
1.7. 1产生误差的原因 7
1.7. 2 分析预测误差的指标和方法 8
1.8负荷预测的现状及存在的问题 9
1.9本章小结 10
[资料来源:Doc163.com]
第二章 电力系统短期负荷预测概述 11
2.1电力系统负荷预测 11
2.1.1电力系统负荷预测类型 11
2.1.2电力系统负荷预测的特点和基本原理 11
2.2电力系统短期负荷预测分析 13
2.2.1电力系统短期负荷特征分析 13
2.2.2电力系统短期负荷中的影响因素分析 13
2.3电力系统短期负荷预测的基本思路 15
2.3.1以安顺市电力系统为例总结短期负荷基本特征 15
2.3.2建立短期负荷预测模型应考虑的问题 16
2.3.3建立短期负荷预测模型基本原则 17
2.4电力系统短期负荷预测中的数据预处理 17
2.5电力系统短期负荷预测误差 18
2.6本章小结 18
第三章 基于人工神经网络模型的电力系统短期负荷预测 19
[资料来源:http://Doc163.com]
3.1引言 19
3.2人工神经网络 19
3.3 B P神经网络 20
3.3.1 BP神经网络结构 20
3.3.2 BP神经网络的学习方式 21
3.3.3 BP算法的数学描述 21
3.3.4 BP神经网络的主要特点 23
3.3.5 BP神经网络的算法流程 24
3.4仿真和预测结果分析 27
3.4.1 BP神经网络的Matlab实现 27
3.4.2 预测模型的仿真过程 28
3.4.3 仿真后的结果分析 31
3.5本章小结 31
第四章 改进的BP神经网络的电力系统短期负荷预测 32
4.1引言 32
4.2 改进的BP神经网络算法: L-M算法 32 [资料来源:http://Doc163.com]
4.3基于L-M算法的模型 34
4.4 仿真结果分析 36
4.5 本章小结 36
第五章 总结与展望 37
5.1 论文研究的主要内容 37
5.2 论文的不足与展望 37
参考文献 39
致 谢 41 [资料来源:www.doc163.com]