半监督学习算法研究
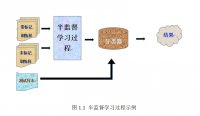
半监督学习算法研究(开题报告,外文翻译,论文13600字)
摘要
时至今日,随着信息技术的迅猛发展,利用计算语言收集有效信息的情况越来越多,人们运用机器语言的地方也就越来越多,收集无标记的样本已经相当容易,而获取有标记的样本因为人力物力的原因则相对困难,机器语言学习领域的三大主要的领域:监督、非监督、半监督,半监督学习能对所有类型的自然样本数据,搭配适当的函数,综合利用再具体分类充分利用未标记样本改善学习性能,对实际情况合理地应对改善,因而,半监督学习依然成为机器学习中的热门。
为了可以有效地解决存在隐含变量问题而提供优化方法,本文想到一种数据添加的经典算法,也就是EM算法。本文目标对象为半监督学习中的EM算法,希望对其进行简单的研究,通过其历史、现状与趋势,了解其发展,阐述其合理性,实用性和可靠性,并且根据算法建立GMM下的Kmeans模型,撰写聚类程序,通过其凹凸变换可以靠谱地找到“最优的收敛值”,观察其在MATLAB上仿真结果,展示在此半监督学习方法下所达到的分类效果,最后得出半监督学习对人类学习发展有着巨大重要性的结论。
当前各类的科学研究以及实际的现状在于应用方面得到巨大的进步,相对而言数据的采集处理等等相关工作量也就越来越大,经容易出现数据缺失、数据错误的的问题,运作工程中,合理地利用好EM算法,可以直接对数据进行附加标签的从而对原始数据进行改善与选择,促进各类学科的研究发展。而随着理论的发展,EM算法己经不仅仅局限于处理数据缺失的问题,人们用它处理的问题日益广泛,对它的学习也就变得尤为重要。
[版权所有:http://DOC163.com]
关键词:无标记;EM;数据缺失;稳定;优化
Abstract
In recent years, with the rapid development of information technology, more and more effective information is collected by the use of computational language, and more and more people use machine language. It is quite easy to collect unlabeled samples, and the acquisition of labeled samples is relatively difficult because of human resources. Machine linguistics is relatively difficult. There are three major fields in the study field: Supervision, unsupervised and semi supervised. Semi supervised learning can match all types of natural sample data with appropriate functions, and make full use of unmarked samples to improve learning performance by using the re specific classification. It's hot for machine learning.
In order to effectively solve the problem of hidden variables and provide optimization methods, this paper thinks of a classical algorithm of data addition, that is, EM algorithm. In this paper, the target object is EM algorithm in semi supervised learning. We hope to do a simple research on it. Through its history, current situation and trend, it understands its development, expounds its rationality, practicability and reliability, and establishes the Kmeans model under the GMM algorithm according to the algorithm, and writes the clustering program, and can be found by its concave and convex transformation. "Optimal convergence value", the simulation results on MATLAB are observed to show the classification results under the semi supervised learning method. Finally, the conclusion that semi supervised learning is of great importance to the development of human learning is concluded.
[资料来源:http://doc163.com]
At present, all kinds of scientific research and actual situation lie in the great progress in the application, and the relative workload of data acquisition and processing is getting bigger and bigger. The problem of missing data and error of data is easy to appear. In the operation project, the EM algorithm can be used properly, and the data can be attached directly to the data. Tagging is used to improve and select raw data and promote research and development of various disciplines. With the development of the theory, the EM algorithm is not only limited to the problem of data loss. People use it to deal with more and more problems, and it becomes particularly important to its learning.
KeyWords: unmarked ;EM ;data missing ;stability optimization
[资料来源:www.doc163.com]
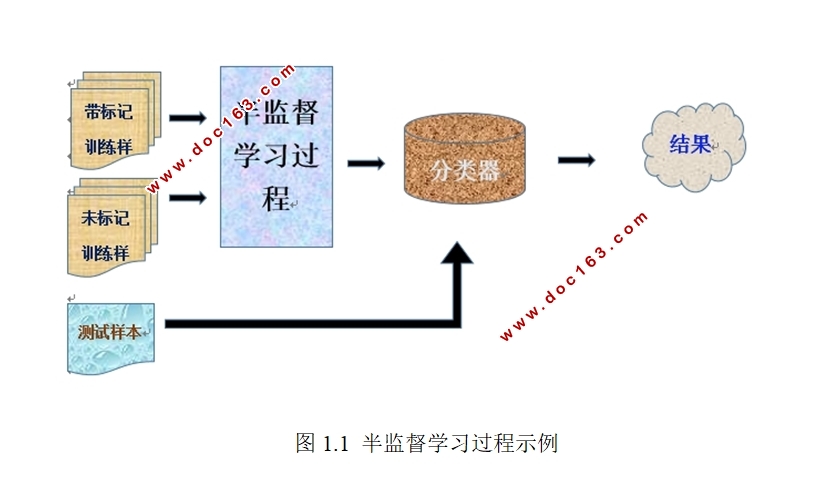
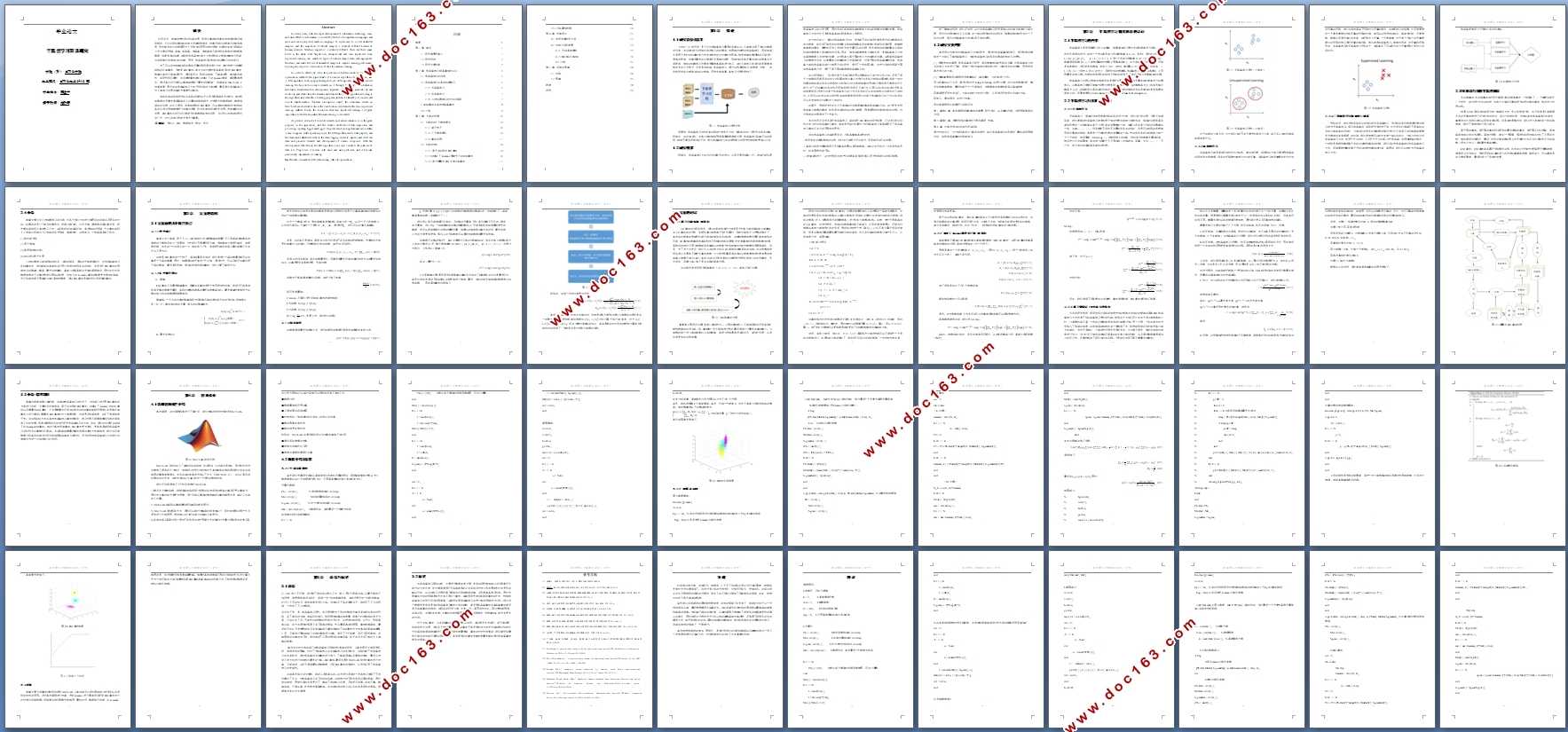
目录
摘要 I
第1章 绪论 1
1.1研究背景及意义 1
1.2研究现状 2
1.3研究主要内容 3
第2章 半监督学习极其算法的认识 5
2.1半监督学习的内容 5
2.2半监督学习与其他机器学习 5
2.2.1无监督学习 5
2.2.2有监督学习 7
2.2.3三种机器学习的对比与联系 7
2.3EM算法与其他半监督算法 8
[来源:http://www.doc163.com]
2.4小结 9
第3章 方案的选择 10
3.1方案前的几个数学要点 10
3.1.1举个例子 10
3.1.2几个数学要点 10
3.1.3算法流程 11
3.2方案的对比 14
3.2.1 基于自训练的EM算法 14
3.2.2 GMM下Kmeans聚类先行的EM算法 16
3.2.3 基于增量式EM文本分类算法 17
3.3小结-最终选择 20
第4章 仿真设计 22
4.1选用仿真软件介绍 22
4.2功能介绍及结果 23
4.2.1生成高斯模型 23
4.2.2 EM解决GMM 26
4.3小结 32
第5章 总结与展望 34
5.1总结 34
5.2展望 35
致谢 38
附录 39
[资料来源:http://doc163.com]